In Brief | Deep Learning Detection of Active Pulmonary Tuberculosis at Chest Radiography Matched the Clinical Performance of Radiologists
Published on MedED: 3 July 2023
Type of article: In Brief
MedED Catalogue Reference: MIID004
Category: Infectious Diseases | TB| Radiology
Sources::Radiology
Published on MedED: 2 July 2023
Google's artificial intelligence (AI) technology may become an essential tool, in improving early tuberculosis (TB) diagnosis, particularly in resource-constrained environments where access to skilled radiologists is limited.
The AI technology, known as DeepMind, has been trained to analyse chest X-rays and detect signs of TB accurately. Researchers of a recent study published in Radiology report that the technology model demonstrated a sensitivity of 94% and a specificity of 97% in detecting TB-related abnormalities, significantly outperforming human radiologists.
The potential benefits of this AI-powered diagnostic tool are significant: by improving the speed and accuracy of diagnosis, it could facilitate early detection, enabling earlier treatment, ultimately leading to better patient outcomes and reduced transmission rates.
The researchers note that several challenges need to be addressed before wide-scale adoption is possible. Further studies and validation are required to ensure its effectiveness across different populations and healthcare settings. Additionally, integrating this technology into existing healthcare systems and ensuring accessibility in regions with limited resources will be crucial.
Access the original article
Kazemzadeh, S., Yu, J., Jamshy, S., Pilgrim, R., Nabulsi, Z., Chen, C., Beladia, N., Lau, C., McKinney, S. M., Hughes, T., Kiraly, A. P., Kalidindi, S. R., Muyoyeta, M., Malemela, J., Shih, T., Corrado, G. S., Peng, L., Chou, K., Chen, P. C., Liu, Y., … Prabhakara, S. (2023). Deep Learning Detection of Active Pulmonary Tuberculosis at Chest Radiography Matched the Clinical Performance of Radiologists. Radiology, 306(1), 124–137. https://doi.org/10.1148/radiol.212213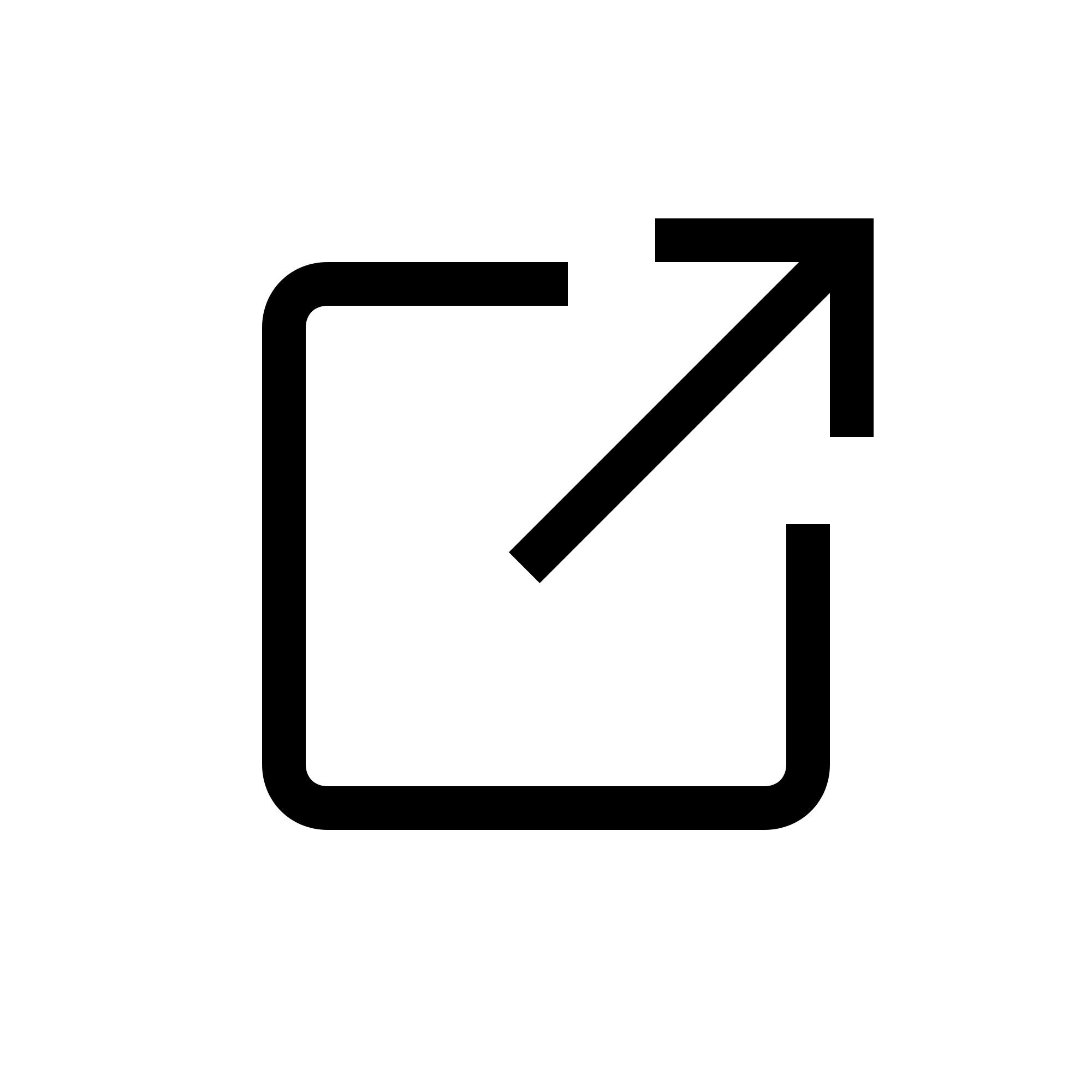
Disclaimer
This article is compiled from a variety of resources researched and compiled by the contributor. It is in no way presented as an original work. Every effort has been made to correctly attribute quotes and content. Where possible all information has been independently verified. The Medical Education Network bears no responsibility for any inaccuracies which may occur from the use of third-party sources. If you have any queries regarding this article contact us
Fact-checking Policy
The Medical Education Network makes every effort to review and fact-check the articles used as source material in our summaries and original material. We have strict guidelines in relation to the publications we use as our source data, favouring peer-reviewed research wherever possible. Every effort is made to ensure that the information contained here is an accurate reflection of the original material. Should you find inaccuracies, out of date content or have any additional issues with our articles, please make use of the contact us form to notify us.